- Research Papers
Ranking Policy Decisions
29 September 2021, 13:54 GMT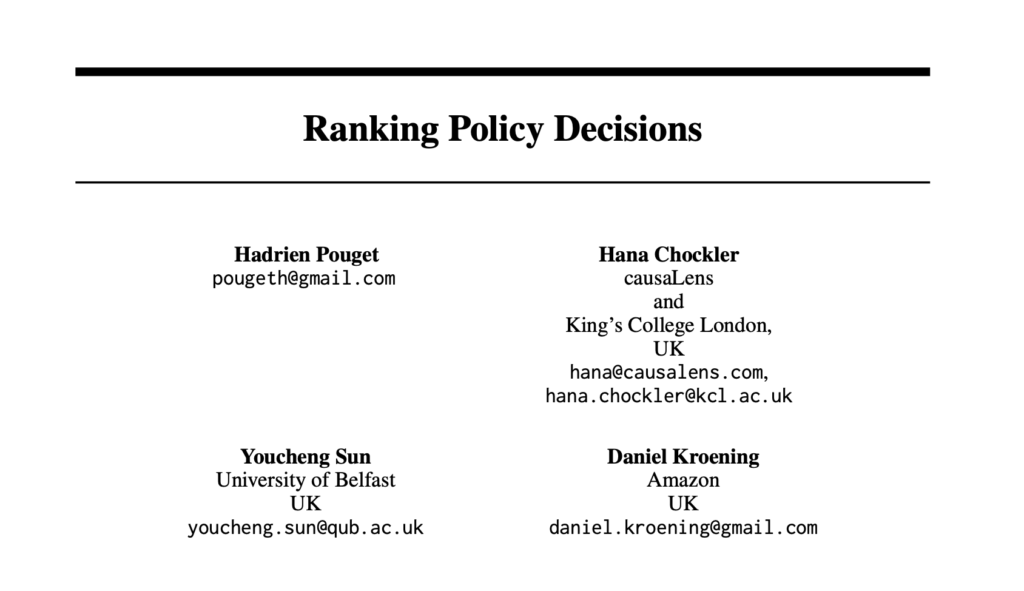
causaLens’ NeurIPS paper introduces a novel method based on root cause analysis to rank states of the environment of a given reinforcement learning policy according to the importance of decisions made in those states. The ranking is used to explain RL policies and to create new, simpler policies by pruning the lower-ranked decisions.
Our own Hana Chockler’s paper “Ranking Policy Decisions” has been accepted to NeurIPS, in collaboration with colleagues from Amazon Science. The paper introduces a novel method, based on causality-enabled fault localization, to rank states of the environment of a given reinforcement learning policy according to the importance of decisions made in those states. The ranking is used to explain RL policies and to create new, simpler policies by pruning the lower-ranked decisions. These simpler policies perform on a level comparable to the original, needlessly complex policies.